EconomicDynamics Research Agenda
Volume 21, Issue 2 (November 2020)
Ben Moll on the Rich Interactions between Inequality and the Macroeconomy
Watch Teaser Video!
Ben Moll is a Professor of Economics at the London School of Economics. His research is concerned with understanding why some countries and people are so much poorer than others and how micro heterogeneity impacts the macro economy and macroeconomic policy. Moll’s RePEc/IDEAS profile.
Introduction
One of the key developments in macroeconomics research over the last three decades has been the incorporation of explicit heterogeneity into models of the macroeconomy. As a result of taking micro data seriously, these theories study macroeconomic questions in terms of distributions of microeconomic variables like income or wealth rather than just aggregates. This approach is attractive for two reasons. First, empirically, it provides an integrated framework for making use of both micro and macro data. Second, conceptually, it provides a kind of “distributional macroeconomics” perspective, meaning an integrated perspective for analysing the distributional implications of macroeconomic trends, shocks or policies and the two-way interaction between distribution and the macroeconomy. My current research aims to contribute to this broader agenda.
The summary below describes some of my research projects in this area. These range from analysing the effects of policies like monetary policy or lockdown measures to tackle COVID-19 (Section 1) to studying potential drivers of rising income and wealth inequality like automation (Section 2) to the development of new methods for thinking about heterogeneity in macroeconomics (Section 3). I will conclude with what I view as some interesting open questions and avenues for future research (Section 4). All of the work I review here reflects collaborations – often over many years – with Yves Achdou, SeHyoun Ahn, Felipe Alves, Paco Buera, Andreas Fagereng, Xavier Gabaix, Jiequn Han, Martin Holm, Greg Kaplan, David Laibson, Jean-Michel Lasry, Pierre-Louis Lions, Peter Maxted, Gisle Natvik, Galo Nuño, Lukasz Rachel, Pascual Restrepo, Gianluca Violante, Tom Winberry, and Christian Wolf.
Writing this summary also prompted me to look back at some of the older RED research agenda pieces that can be found here. I highly recommend you do the same because they provide a very nice insight into the historical development of the agenda I described above, namely to develop an integrated approach to macroeconomic and distributional considerations as well as macro and micro data. See in particular the pieces by Victor Rios-Rull (2001), Tony Smith (2003), Kjetil Storesletten (2003), and Dirk Krueger and Fabrizio Perri (2005). Many of the themes in my research summary – as well as, more broadly, many of the recent developments in heterogeneous-agent macroeconomics – follow logically from the visions set out in these earlier pieces.
1. HANK Models for Macroeconomic Policy Analysis
Primarily in collaboration with Greg Kaplan and Gianluca Violante, one of the main themes of my work has been the development of richer and more empirically realistic models for macroeconomic policy analysis. These Heterogeneous Agent New Keynesian (HANK) models combine features from the heterogeneous agent (HA) literature, namely heterogeneity and incomplete markets, and the New Keynesian (NK) literature, namely nominal rigidities. They open the door to studying distributional issues, business-cycle fluctuations, and stabilization policies, all within the same framework.
In “Monetary Policy According to HANK” (Kaplan, Moll and Violante, 2018) we revisit the transmission mechanism from monetary policy to household consumption in such a model, calibrated to yield empirically realistic distributions of wealth and marginal propensities to consume. In standard Representative Agent New Keynesian (RANK) models, monetary transmission is based almost entirely on intertemporal substitution. In contrast, in our HANK model this channel is small. Monetary policy nevertheless has sizeable real effects because of indirect effects, in particular those operating through a general equilibrium increase in labor demand and disposable incomes of high-MPC households. In Alves, Kaplan, Moll and Violante (2020) we follow up this work by examining in more detail the relative importance of different HANK model elements (e.g. unequal incidence of aggregate income fluctuations across households or the distribution of profits) for amplification or dampening of the response of aggregate consumption to a monetary shock.
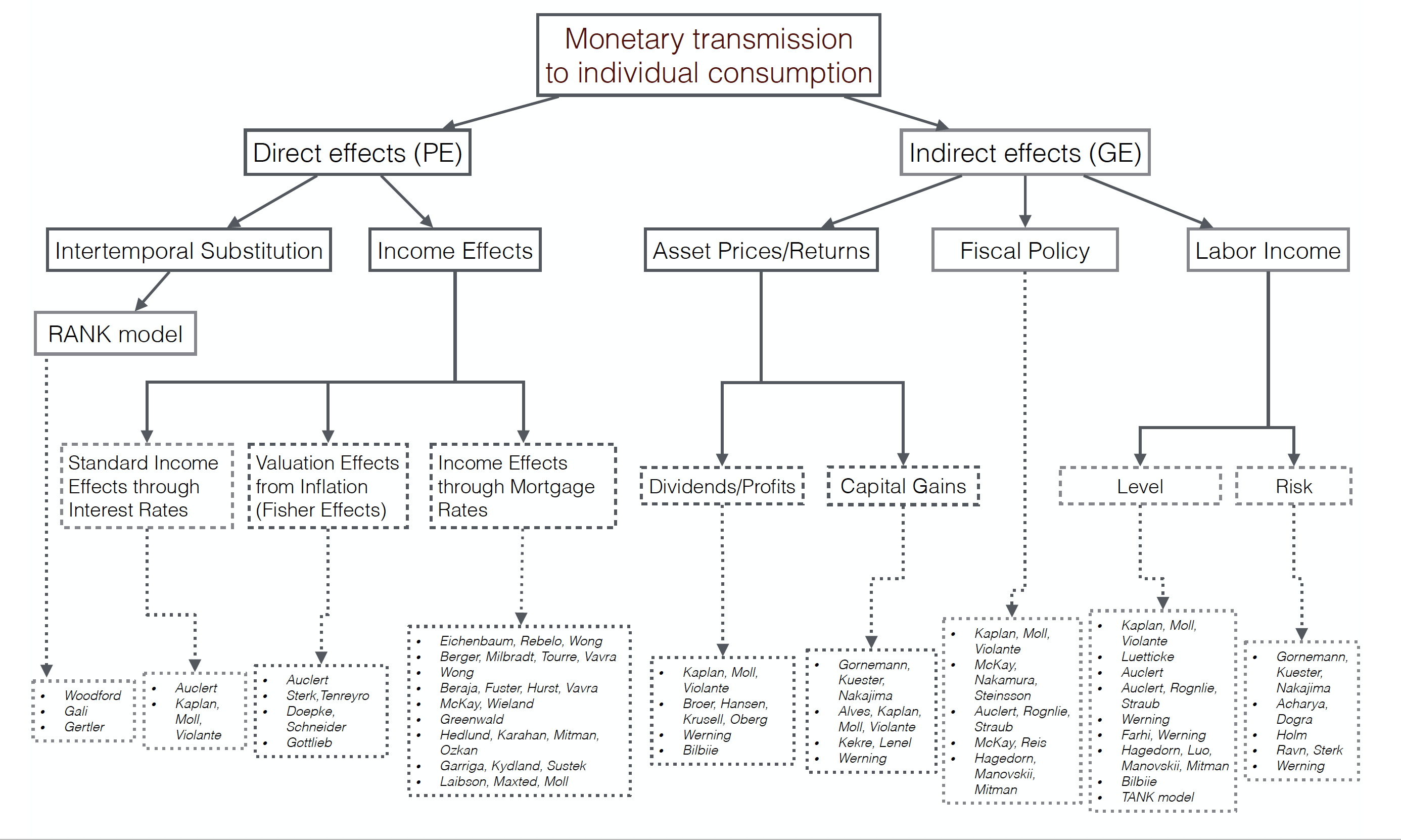